How AI is Shaping the Future of Drug Discovery and Chemistry
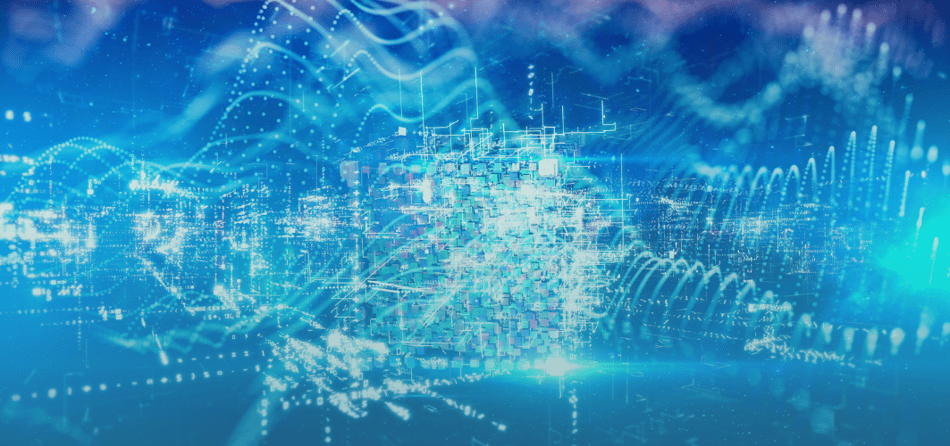
Artificial Intelligence (AI) is already having a major impact on the pharmaceutical industry, and it has the potential to revolutionize medicinal chemistry and unlock new possibilities in drug discovery.
Dr. Kevin Hunt, Chief Scientific Officer at Vanqua Bio, brings decades of experience to the intersection of drug development and cutting-edge technologies like AI. With over 50 patents and publications, and a track record of discovering clinical candidates for life-threatening diseases, Dr. Hunt has witnessed firsthand how AI is reshaping the research landscape.
"I think within the next five years, AI will be on the desk of most medicinal chemists, and not just as a tool, but as an essential desktop app," he said, highlighting the growing role AI plays in the daily workflows of researchers.
We recently spoke with Dr. Hunt to learn more about current applications of AI, the challenges it faces, and the pivotal role that Contract Development and Manufacturing Organizations (CDMOs) play in leveraging this technology for pharmaceutical innovation.
The Evolution of AI in Chemistry
“Machine learning has been used in chemistry for decades,” Dr. Hunt noted, emphasizing the long-standing relationship between AI and the field. This connection began in the 1960s with the DENDRAL (DENDRitic ALgorithm) project at Stanford University, which automated the identification of unknown organic molecules by analyzing mass spectra and chemical data.
DENDRAL not only showcased AI's ability to tackle complex scientific problems but also laid the groundwork for knowledge-based systems in chemistry, proving that AI could effectively manage tasks requiring specialized expertise.
From there, AI's role in chemistry and related fields expanded steadily, including:
- 1970s-1980s: Expert systems like MYCIN emerged, extending AI's reach into medical applications and drug design. MYCIN, also developed at Stanford, was used for diagnosing blood infections and recommending antibiotics
- 1980s-1990s: Quantitative Structure-Activity Relationship (QSAR) models used statistical methods to correlate chemical structures with their biological effects, greatly accelerating the drug discovery process
- Late 1990s-2000s: Machine learning techniques like support vector machines and random forests began to be applied to chemical problems, offering improved predictive power over traditional statistical methods.
- 2010s-present: Deep learning and neural networks have revolutionized chemical predictions, offering unprecedented insights into molecular structures and interactions.
Recent years have seen a shift from centralized, hardware-intensive computing to more accessible platforms. “The newer AI models allow you to do great things with your PC, offering more opportunities with much less heavy lifting,” Dr. Hunt explained. This democratization of tech has lowered entry barriers for smaller research teams, potentially accelerating drug discovery.
He noted, however, that cutting-edge technology still needs to be balanced out with proven methodologies – at least for the time being. “I still tend to use more traditional machine learning, as I find the data more predictive,” he said.
How AI is Revolutionizing Drug Discovery: Key Applications and Benefits
As AI continues to evolve, its applications in drug discovery are becoming increasingly sophisticated and impactful, including:
- Enhancing patient population analysis
One of the most promising applications of AI in drug discovery is its ability to analyze vast amounts of data to identify and characterize patient populations.
“AI is great for sifting through publications, finding connections in data from patents and research, and bringing together pathways for personalized medicine and genetics,” Dr. Hunt explained. By identifying patterns that human researchers might overlook, AI can help pinpoint specific patient groups most likely to benefit from particular treatments. - Streamlining disease pathway identification
AI's data processing capabilities extend to identifying and mapping disease pathways. By analyzing vast datasets of genetic information, clinical trials, and research, AI helps researchers understand disease mechanisms more quickly and thoroughly.
This accelerated understanding can lead to more targeted drug development, potentially reducing time and costs for bringing new treatments to market. - Reducing human bias in data interpretation
One of the key advantages of AI in data analysis is its ability to approach information without human biases.
“AI does that better than humans do, in my opinion,” Dr. Hunt said, “if you feed it the right data set.”
This unbiased approach can uncover important connections or potential treatments that might be overlooked due to preconceived notions. - Potential impact on personalized medicine
AI's potential to accelerate personalized medicine development is one of its most exciting applications in drug discovery. By analyzing individual patient data alongside vast genetic and treatment outcome databases, AI can identify the most effective treatments for specific patient profiles, significantly improving outcomes and reducing trial-and-error.
“That's where it's at for me,” Dr. Hunt said. “I think AI’s capacity to analyze and connect data sets and published information and disease pathology from diverse sources is incredibly valuable in this context.”
AI in Drug Discovery: Overcoming Challenges and Limitations
While AI's potential in drug discovery is immense, it faces several challenges:
- Complexity of molecular interactions
A primary challenge is the inherent complexity of molecular interactions. "Making a drug is all about three-dimensionality," Dr. Hunt noted, highlighting the difficulty in accurately predicting molecular interactions in 3D space.
AI models, especially those based on 2D molecular representations, often struggle to capture this complexity, leading to predictions that may not translate well to real-world drug behavior. - Data quality and availability hurdles
The effectiveness of AI models is heavily dependent on the quality and quantity of data they're trained on, and as Dr. Hunt pointed out, “The quality of the data coming in is often poor or not well reported."
This – which can include inconsistent reporting standards, incomplete datasets, and errors in published literature – can severely hamper the predictive power of AI models. - The data advantage of large pharmaceutical companies
“Large pharmaceutical companies have decades of data from previous drug development efforts to feed the beast and train robust AI models,” Dr. Hunt explained.
This data advantage could widen the gap between big pharma and smaller players, potentially impacting industry competition and innovation. - Intellectual property concerns
The use of AI in drug discovery raises complex questions about IP rights. For instance, if an AI system identifies a novel drug candidate, who owns the rights to that discovery? How can companies protect their proprietary data when using third-party AI tools or participating in data-sharing consortiums?
These are massive questions to answer, as “If you don't have your IP, you don't have anything in terms of drug discovery and development,” Dr. Hunt emphasized. - Potential workforce changes
AI's integration into drug discovery may shift required industry skills, creating new opportunities in AI development and pharmaceutical data science but also potentially displacing some jobs.
Managing these transitions thoughtfully will be crucial, ensuring human expertise complements AI capabilities for optimal results.
Maximizing AI Potential: Partnering with CDMOs in Drug Development
CDMOs are uniquely positioned to tackle challenging problems in drug discovery and development using AI, helping you:
- Solve complex problems
By combining their extensive expertise with cutting-edge AI technologies, CDMOs like GL CHEMTEC can address complex issues and pharmaceutical challenges that might be beyond the scope or capabilities of individual pharmaceutical companies.
“Quality CDMOs like GL CHEMTEC don't get the easy problems,” Dr. Hunt said. “They solve problems other people haven't thought of, things like “What's a polymer we should consider that we haven't?” - Enable less experienced research teams
Partnering with tech-savvy and AI-powered CDMOs can help bridge the gap between novice researchers and seasoned experts by providing data-driven insights and suggestions. This democratization of expertise allows smaller biotech companies and startups to tackle complex problems that might have previously been out of reach. - Foster collaborative AI efforts and data sharing
CDMOs are well-positioned to facilitate collaborative efforts in AI and data sharing. By acting as neutral third parties, they can aggregate data from multiple sources, ensuring that AI models are trained on diverse and comprehensive datasets.
“CDMOs can play a crucial role in bringing together dozens of companies to pool our data for training AI systems,” Dr. Hunt explained. “This collaborative approach allows us to create more robust prediction models based on larger, more diverse datasets. It's a win-win situation where everyone benefits from the collective knowledge while maintaining their individual competitive edge.” - Balance artificial and human intelligence
While AI offers powerful capabilities, Dr. Hunt emphasizes the importance of balancing artificial intelligence with human expertise. CDMOs like GL CHEMTEC don't just provide AI tools; they offer a combination of cutting-edge technology and deep industry experience.
“When I reach out to a partner, I'm looking for someone who understands where I need help and can key in on the problem,” Dr. Hunt said. “If they have AI helping them, that’s fantastic. But with companies like GL CHEMTEC, you’re also getting real-world problem-solving experience. Artificial intelligence is great, but combining it with real intelligence is even better.”
GLC: Your Trusted CRO/CDMO Partner
GL CHEMTEC is passionate about your success and committed to solving your most complex chemistry challenges. We offer fast, flexible, cutting-edge solutions to take your medicinal chemistry and early stage small molecule routes to the next level. Our commitment to a collaborative partnership means we scale and adapt precisely to meet your evolving needs.
GL CHEMTEC provides:
- A secure North American alternative to overseas providers, offering R&D labs, Kilo labs and scale-up facilities in the Greater Toronto area
- A local partner who will ensure secure shipping, real-time communication and the highest levels of IP protection for your early stage work
- A highly customer-centric culture with a 20+ track record of success
- ISO 9001:2015 and ISO 13485:2016 certifications
- A GMP Cleanroom with GMP Reactor Suites coming online soon